In recent years, the financial markets have witnessed a significant transformation, largely fueled by advancements in artificial intelligence (AI). This technological evolution has reshaped how market analysts, traders, and financial strategists view and interact with market data. AI’s integration into financial markets represents a paradigm shift from traditional analytical methods towards more dynamic, efficient, and predictive modeling capabilities. This article aims to delve deeply into how AI is revolutionizing stock market predictions. We will explore the powerful benefits AI brings to financial analytics, address the challenges it presents, and assess the impact of AI technologies on the future of trading and investment strategies.
Understanding AI in Financial Contexts
Definition of Artificial Intelligence and Its Financial Applications
Artificial Intelligence, in the context of finance, refers to the capability of machines or programs to perform tasks that typically require human intelligence. These tasks include reasoning, generalization, recognition, and problem-solving. In the financial sector, AI is primarily applied to predict market trends, manage investment portfolios, and for risk management. These applications are made possible by AI’s ability to rapidly analyze large datasets and make predictions based on identified patterns.
How AI Differs from Traditional Statistical Models
Unlike traditional statistical models that often linearly interpret data and require manual intervention for updates, AI introduces models that can learn from data in an iterative and sometimes autonomous manner. This allows AI to adapt to new information and changing market conditions more effectively than traditional models. AI systems can analyze not only numerical data but also unstructured data such as news articles, social media posts, and even speeches to gauge market sentiment or potential political impacts on the markets.
Overview of AI Technologies in Financial Analytics
AI technologies that have made a significant impact in financial analytics include machine learning (ML), neural networks, and deep learning. Machine learning algorithms improve their performance as the number of datasets available for learning increases. Neural networks, which are designed to mimic human brain operation, are particularly effective in recognizing patterns and making predictions. Deep learning, a subset of ML, utilizes multi-layered neural networks and has proven exceptionally powerful in handling vast amounts of data with complex relationships and hierarchies. These technologies enable financial models to perform tasks ranging from fraud detection and risk assessment to asset price prediction and algorithmic trading with unprecedented speed and accuracy.
Evolution of AI in Stock Market Predictions
Historical Perspective on Technology in Stock Trading
The journey of technology in stock trading and analysis has been one of constant evolution, from the days of ticker tapes in the early 20th century to the complex computer algorithms used today. Initially, technology was used primarily for the execution of trades and data management, but as computers became more powerful, their role expanded to include market analysis and prediction.
Key Milestones in AI Integration
The integration of AI into market predictions marks a significant milestone in financial technology. In the 1980s, the introduction of algorithmic trading represented one of the first major uses of computational tools to automate trading strategies based on predefined criteria. By the late 1990s and early 2000s, advancements in machine learning began to allow for more sophisticated analyses of patterns and trends in stock market data. This period also saw the development of neural networks capable of simulating human decision-making processes, further enhancing the predictive capabilities of financial models.
Case Studies of Early Adopters
One notable early adopter of AI in financial analysis was Renaissance Technologies, founded by mathematician James Simons. Renaissance developed complex mathematical models that successfully predicted price changes in financial instruments. Another example is Goldman Sachs, which has invested heavily in AI for various financial tasks, including trading. These institutions recognized the potential of AI to transform financial analysis and have reaped significant benefits from its implementation.
How AI Enhances Market Predictions
AI in Data Processing for Predictions
AI algorithms excel at processing and analyzing vast amounts of data rapidly and with high accuracy. They can examine large datasets — beyond what human analysts could feasibly handle — identifying complex patterns and correlations that inform predictive models. This capacity is critical in today’s global markets, where the volume and velocity of data continuously grow.
Capabilities in Pattern Recognition and Predictive Analytics
AI’s capabilities extend into pattern recognition, anomaly detection, and predictive analytics. These technologies allow AI systems to identify profitable trading opportunities, detect deviations from typical market patterns that may indicate potential market shifts, and predict future market behaviors with a high degree of accuracy. For example, AI systems can analyze news feeds in real-time to assess market sentiment and incorporate these insights into predictive models.
Examples of AI Predicting Market Dynamics
AI has proven its worth in predicting market trends, crashes, and recoveries. During the 2008 financial crisis, some AI systems identified patterns that hinted at an impending crash, allowing traders to mitigate risks. Similarly, AI-driven models have been used to predict recovery periods and optimal re-entry points into the market following downturns.
Evolution of AI in Financial Market Predictions
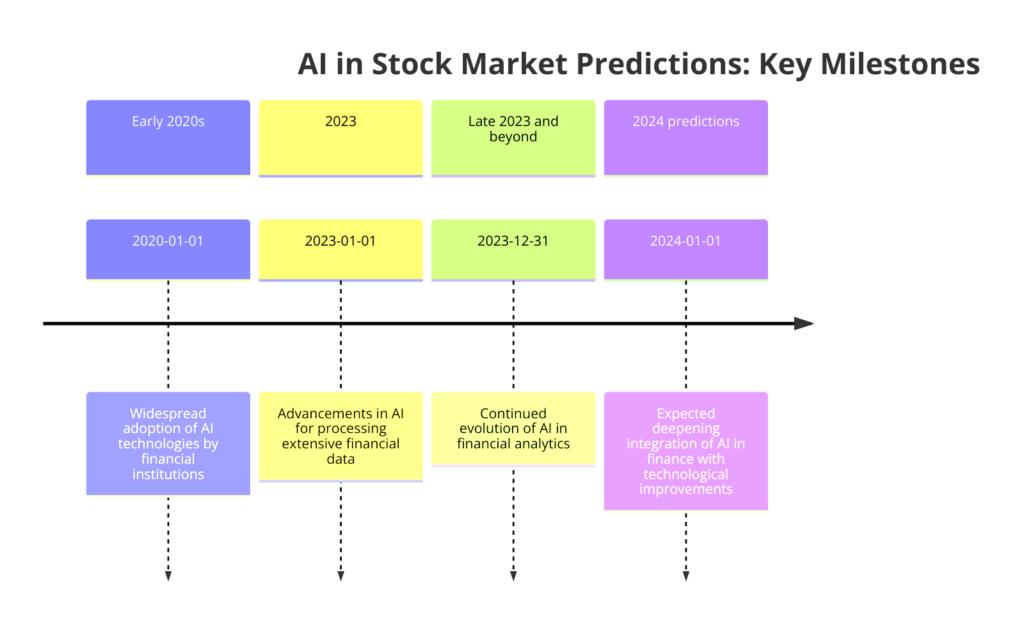
As the financial industry continues to embrace technological advancements, the role of artificial intelligence (AI) in market predictions has become increasingly pivotal. The timeline diagram below encapsulates the significant milestones and developments in AI that have shaped stock market predictions over the recent years.
From the early 2020s, financial institutions have increasingly adopted AI technologies, employing tools such as generative AI, neural networks, and natural language processing. This period marked the beginning of a transformative era in which AI began to redefine the traditional methodologies used in stock market analytics.
The year 2023 witnessed further advancements, with AI enhancing its capability to process and analyze vast amounts of financial data. These improvements have facilitated more sophisticated predictive models that leverage deep learning, sentiment analysis, and reinforcement learning to forecast market trends with unprecedented accuracy.
Looking ahead to late 2023 and beyond, the integration of AI in financial analytics is expected to evolve further. This continued development is geared towards refining AI’s predictive accuracy and reliability, addressing challenges such as data quality and model overfitting.
For 2024 and onwards, AI is anticipated to deepen its integration into the financial sector, driven by technological advancements that promise to expand the capabilities of AI in enhancing market prediction models. This forward momentum is expected to bring about a new era of personalized investment services and more efficient risk management strategies.
AI-Driven Investment Strategies
AI in Developing Investment Strategies
Investors and fund managers increasingly rely on AI to develop and refine investment strategies. AI algorithms can simulate millions of potential scenarios, helping fund managers understand potential outcomes and make informed decisions. This capability is particularly useful in constructing diversified portfolios that can withstand various market conditions.
Impact on Portfolio Management
AI significantly impacts portfolio management by providing tools for real-time risk assessment, scenario analysis, and strategy adjustment. This enables more dynamic asset allocation, which can adapt to market changes more quickly and effectively than traditional methods.
Case Studies in AI Implementation
Many hedge funds, such as Two Sigma and Bridgewater Associates, have integrated AI into their investment strategies to great effect. These funds use AI to analyze multiple data sources for insights into market movements and to automate large portions of the trading process. Investment banks like J.P. Morgan have also developed proprietary AI tools to aid in trading, risk management, and customer service, highlighting the broad applications and trust in AI technologies in the financial sector.
Challenges and Limitations of AI in Stock Predictions
Limitations of AI in Financial Markets
While AI offers transformative potential in stock market predictions, it is not without its limitations. One major concern is data quality. AI systems are only as good as the data they process, and inaccurate or biased data can lead to misleading predictions. Additionally, overfitting remains a significant challenge; this occurs when an AI model is too closely fitted to the historical data it was trained on, making it less effective at predicting future, unseen outcomes. Another issue is model interpretability, often referred to as the “black box” problem, where the decision-making process of AI algorithms is not easily understood by humans, making it difficult to trust or evaluate the reasoning behind AI decisions.
Ethical Considerations and Dependency Risks
The increasing reliance on AI for financial decision-making also raises ethical concerns. There is a risk that dependency on automated systems could lead to a lack of human oversight, potentially exacerbating financial crises if AI systems react unexpectedly to market conditions. Ethical considerations also include the potential for AI to unintentionally discriminate based on flawed data inputs, leading to unequal treatment of individuals or companies in the financial markets.
Regulatory Challenges
Deploying AI in financial predictions also involves navigating a complex regulatory landscape. Regulators are still catching up with the rapid advancements in AI technology, leading to a lack of clear guidelines and standards for the use of AI in finance. This uncertainty can inhibit the adoption and development of AI technologies due to concerns about compliance and potential legal liabilities.
The Future of AI in Financial Markets
Future Integration of AI in Finance
The future of AI in financial markets looks promising, with expectations of deeper and more sophisticated integration. AI is anticipated to become more adept at handling complex predictive tasks with greater accuracy and speed, potentially leading to more personalized investment services and more efficient risk management.
Innovations on the Horizon
Upcoming innovations in AI are expected to further transform stock market predictions. Advancements in neural networks and deep learning could lead to even more powerful models capable of understanding and analyzing vast amounts of data from diverse sources, including social media, economic reports, and global news. Enhanced natural language processing abilities will improve AI’s understanding of human language, allowing for more accurate sentiment analysis and its implications for market movements.
Balancing AI and Human Expertise
Despite AI’s advancements, the balance between human expertise and automated technology remains crucial. The nuanced understanding and emotional intelligence of human professionals complement AI capabilities, especially in unpredictable or unprecedented scenarios. This synergy will continue to shape the optimal strategies for financial decision-making.
In Conclusion
Throughout this discussion, we’ve explored how AI is reshaping stock market predictions, unveiling both the remarkable benefits and the significant challenges. The journey of AI integration into financial markets underscores the need for a balanced approach that leverages both advanced technology and human insight. As we look forward, it is clear that continuous adaptation and embracing of AI technologies are vital for maintaining competitiveness and operational efficiency in finance. Encouraging ongoing research and development in AI will be crucial to enhancing its reliability and effectiveness, ensuring that AI not only evolves as a tool for financial predictions but also operates within an ethical, understandable, and regulated framework.